Everything you need to know about developing accurate and reliable sales forecasts
If you are reading these lines, it is because you want to know everything about sales forecasting and most certainly that you are looking to optimize your current method or to implement your very first prediction.
However, if you are here, it is because you have understood the interests and the stakes of sales forecasting for the performance and the profitability of your company (efficient management of logistics, and in particular of stocks, assortment planning, anticipation of customers' behaviors, etc.).
As we will see, in addition to the choice of an adapted sales forecasting solution, the choice of the predictive method is also important, because it will determine the accuracy and reliability of your forecasts and consequently the results of the various actions taken.
Practical, technical and financial aspects, we explain you all you need to know to elaborate a forecasting strategy adapted to your company and its activity sector.
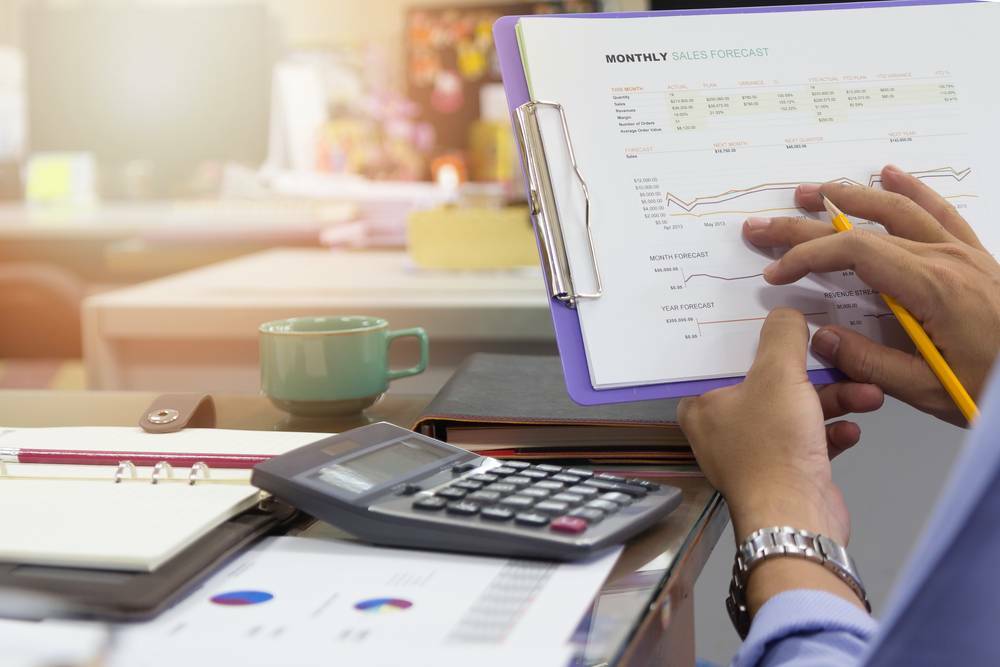
Choose the right sales forecasting method for your company
Implementing a sales forecast is essential to have a 360° vision of your company's activity in a market evolving constantly, but choosing the most appropriate method for your company's own operation and specific sector of activity is just as important.
It is important to understand that each company is unique, even within the same sector, especially in terms of:
- resources (material, financial, human, technical, etc.);
- objectives and ambitions (process optimization, revenue gain, cost reduction, etc.);
- past experiences (data history);
- values (human, proximity, environmental, etc.);
- organization (online sales, single store, several sales outlets, etc.);
- etc.
That's why the wind forecasting method used by a competitor may not be the right answer for your business.
There are several different sales forecasting methods. Although they are all based on the analysis of historical data, they all differ in the information they incorporate into their forecasting models, such as incorporating sales cycles into the determination of a probability, taking into account the intuition of sales teams and their knowledge of customers, analyzing several variables from both historical data and information from the company's environment, etc.
None of these methods is better or worse than another, as long as it is chosen with knowledge of its advantages and disadvantages and in correlation with the company's needs, work habits and budget.
"Its budget"? Because making sales forecasts is expensive? The answer to these questions is yes and no.
Yes, because some methods (such as multi-variable analysis) require the use of advanced sales forecasting software, i.e. incorporating artificial intelligence (ultra-powerful algorithms and machine learning). No, because other methods can be implemented with a simple Excel spreadsheet (although we advise against it) or CRM software (more limited, but sufficient for some companies).
In short, each case has its own adapted method.
Take into account the factors impacting the sales forecast
One of the questions that many business leaders or marketing managers ask themselves is how to improve sales forecasts that are sometimes too inaccurate and, in the worst case, unreliable.
So, whether it's a question of calculating a global sales forecast, forecasting the sales of a specific product or even calculating sales according to a given period (month, quarter, year, etc.), the common point of all these objectives is the impact that certain factors, both internal and external, can have on the sales assumptions.
A company is constantly evolving, just like its market and its environment. How can we expect to make reliable forecasts if these changes are not taken into account? All forecasting methods are based on the analysis of historical data, i.e. the company's past experiences, but few of them include future changes, which are numerous.
These significant changes can come from the company itself, such as human resources management (recruitment, training, end of contract, long-term sick leave, retirement, etc.) or supply chain management, a change in service providers or new equipment for example.
But above all, it can be a question of unknown and unwanted evolutions coming from the direct environment of the company, such as:
- competition (new competitor in the immediate vicinity, change in pricing policy, large-scale commercial operation, new product, etc.);
- the market (loss of demand for a product, change in customer consumption habits, new needs, etc.);
- strategic factors specific to each sector of activity (weather, seasonality, road traffic, etc.);
- the economic, social and regulatory context, etc., such as the effects of the Covid-19 health crisis.
When a company's sales forecasts depend on many factors, the use of forecasting software using machine learning can be the key to accurate and reliable predictive models. In addition to allowing the integration of a large number of factors impacting the business in the forecasting calculation, this type of tool also allows to highlight the major trends to come, information that is crucial for the positioning of the company on its market and its ability to adapt.
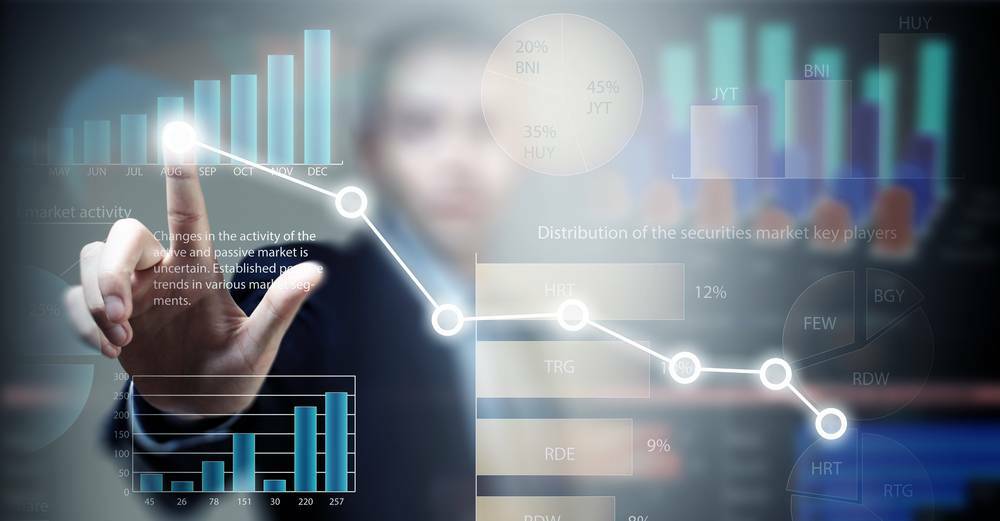
Adapt the analysis and data to the defined objective
There is a lot of data in a company, especially when it comes to sales forecasts. As we have just explained, in addition to the historical data of the business, the forecasting calculation must take into account the external environment, of which we have given you a small overview in the previous section.Nevertheless, although a sales forecast is more reliable and accurate when it integrates a plurality of factors and variables, one thing should be kept in mind: not all data have the same utility and do not serve the same purpose.
Indeed, making forecasts in a business plan does not require the same analysis and the same information as a forecast intended for marketing purposes. In the first case, the elaboration of the financing plan and the cash flow plan requires an analysis of data oriented around financial and economic variables, such as the realized turnover, the critical turnover, the desired evolution, or the different profitability margins (safety margin, margin on variable expenses, etc.).
In the second case, the data analysis focuses on more precise information and variables, such as:
- products (category, price, brand, packaging, etc.);
- the points of sale (location, sales area, inventory, average turnover, etc.);
- the sales team (sales quotas, experience, etc.);
- the sales channel (points of sale, e-commerce, etc.).
Developing a reliable, accurate and, above all, useful sales forecast for strategic decision-making must serve a specific objective.
Ensure the reliability and relevance of the data
How can we end this guide to developing an accurate and reliable sales forecast without taking a look at the pitfalls of sales forecasting? Because there are plenty of potential traps, believe us.Since data analysis is the only source of information for sales forecasting, any missing, truncated or erroneous information has important consequences on the forecast. The best example we can give you, and one that is more common, is out-of-stocks.
Most companies do not track out-of-stocks, so this information is missing from the forecast calculation. Whether the model is elaborated by Man or a CRM software, the result remains the same: the sales not realized because of lack of stock on the product are not taken into account!
This fact, which is very well known, even has a name: the downward bias. Indeed, if a product has been sold in so many copies (available stock before it is sold out), then we can expect to sell as many next month, or even more. Except that in reality, the company might have sold twice or three times as many if the stocks had been higher or replenished. The sales forecast on this product is therefore distorted.
The relevance and reliability of the data are therefore essential, because at the scale of the company and its various sub-activities, the possible errors are numerous. The reliability of the information is also based on its real time update, if necessary on a daily basis, such as data feedback from the field teams or the points of sale, but also by the realization of regular inventories to avoid phantom stocks.
But fortunately, with machine learning-based sales forecasting software, companies have at their disposal tools that allow them to better manage their inventory (in order to avoid stock-outs, for example, by better anticipating customers' needs), as well as the possibility to detect possible errors and other anomalies in the predictive models.
Making a sales forecast is not something that can be improvised. It has to be thought through and designed with regard to the objectives pursued, the method used, the resources and the information available.
We recommend these other pages: